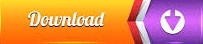

You must be a licensed user of FMPerception with up-to-date support in order to install FMComparison. FMComparison is currently not licensed independently from FMPerception.I would love to get confirmation of that from users.
Geist 2 installer windows#
I’ve only tested in Windows 10, but it should work back to Windows 7. Exercise caution when using the results to guide development decisions. I can make no guarantees about the accuracy or completeness of the results until it has been fully validated by myself and by user experience. Used with permission from Microsoft.You should absolutely read all of the following before using FMComparison.ĬAVEAT: This is beta software. To learn about input variable correlation methods in Simulation Master, check out this article.Įxcel is a registered trademark of Microsoft Corporation. Also, there could also be a shift in the outcomes to the left or right.Īre you looking for a Monte Carlo simulation add-in packed with features at a reasonable price? If so, visit the Simulation Master product page.
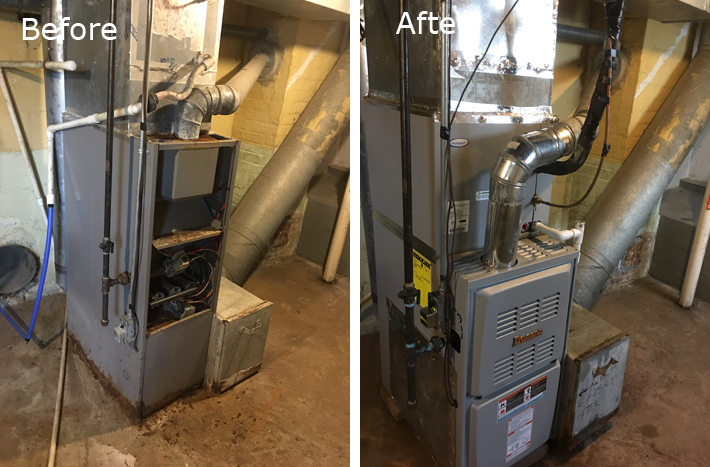
By assuming independence of inputs, we could be under or over estimating risk. We've looked at three examples where input variable correlation affects the outcome differently. In this example if we assumed independent inputs, we would have underestimated risk.įinal Thoughts on Input Variable Correlation Note that the mean of each simulation is nearly Output range with correlated inputs: 26.87 to 78.96 Output range with independent inputs: 29.65 to 73.32 In the first simulation we will assume that demand and price are independent.Īfter running the simulations we see that there is more risk in the correlated simulation. For price we will assume a triangular distribution with 125 as worst case, 150 as most likely, and 190 as best case. For demand, we will assume a triangular distribution with 10 as worst case, 20 as most likely, and 35 as best case. We will run two simulations to compare independent inputs Of course, knowing if correlation is present may be a difficult question to answer, but for our example we will assume we know the correlation. When price increases, demand decreases and vice versa. Demand and price are negatively correlated. We will consider three simple examples to illustrate how input variable correlation affects simulation output.Ĭonsider a very simple model of revenue that has demand and price as inputs. For negatively correlated inputs, one should be at the high end of possible values while the other should be at the low end for a given iteration.
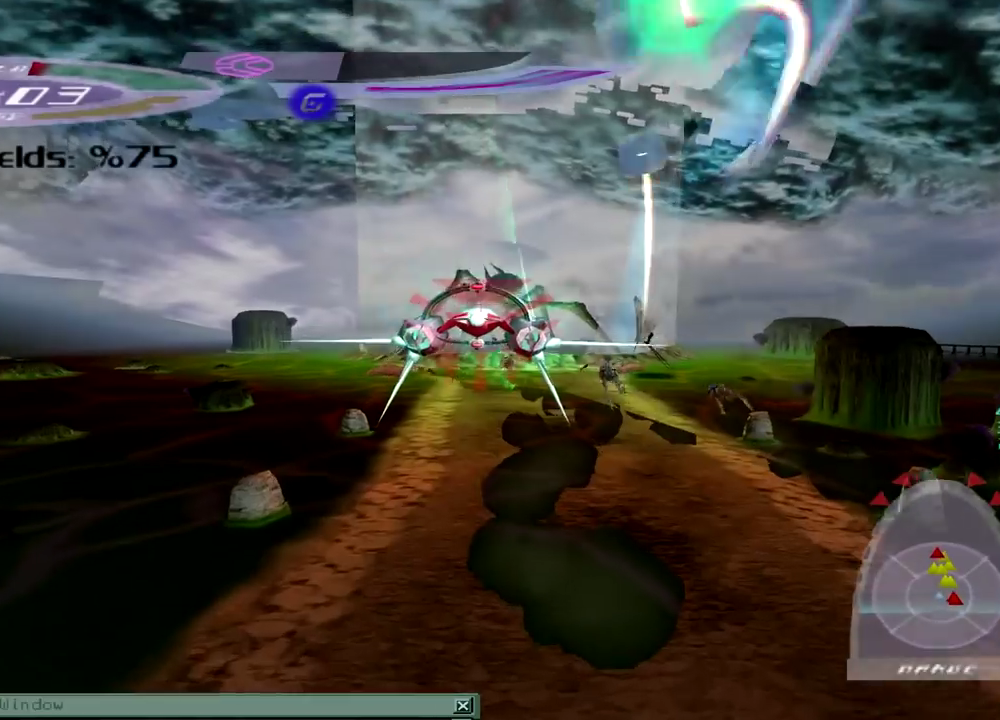
How this occurs, when two input variables have positive correlation, the value for each should be relatively high in a given simulation iteration and both relatively low in another iteration. If input random variables are treated as independent, when they are actually correlated, risk can be under or over estimated. When conducting a Monte Carlo simulation, correlation among input variables is an important factor to consider. ppfs is passed as a list of functions which each have one input and one return value. np.random.multivariate_normal does a lot of the heavy lifting for us, note that in particular we do not need to decompose the correlation matrix. Np.set_printoptions(suppress=True) # suppress scientific notationĪ few note on this function. both percentiles matchį2 = run_gaussian_copula_simulation_and_get_samples( both distributions are independentį1 = run_gaussian_copula_simulation_and_get_samples( Return np.array((U) for i in range(num_dims)]).T # shape($num_samples, $num_dims) # Each row of the returned array will represent one random sample -> access with a # Apply ppf to transform samples into the desired distribution # Transform back into a uniform distribution, i.e. Ran = np.random.multivariate_normal(np.zeros(num_dims), cov_matrix, (num_samples,), check_valid="raise") # Draw random samples from multidimensional normal distribution -> shape($num_samples, $num_dims) Num_samples: int, # number of random samples to draw Ppfs: typing.List, np.ndarray]], # List of $num_dims percentile point functionsĬov_matrix: np.ndarray, # covariance matrix, shape($num_dims, $num_dims) Def run_gaussian_copula_simulation_and_get_samples(
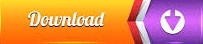